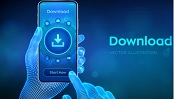
If you have hundreds of images, you would be better running that in parallel which would be: parallel magick mogrify -resize 128x128\! ::: *.jpg
#OPENCV RESIZE WINDOWS#
I would suggest GNU Parallel if you have lots of things to be done in parallel and even more so as CPUs become "fatter" with more cores rather than "taller" with higher clock rates (GHz).Īt its simplest, you can use ImageMagick just from the command line in Linux, macOS and Windows like this to resize a bunch of images: magick mogrify -resize 128x128\! *.jpg
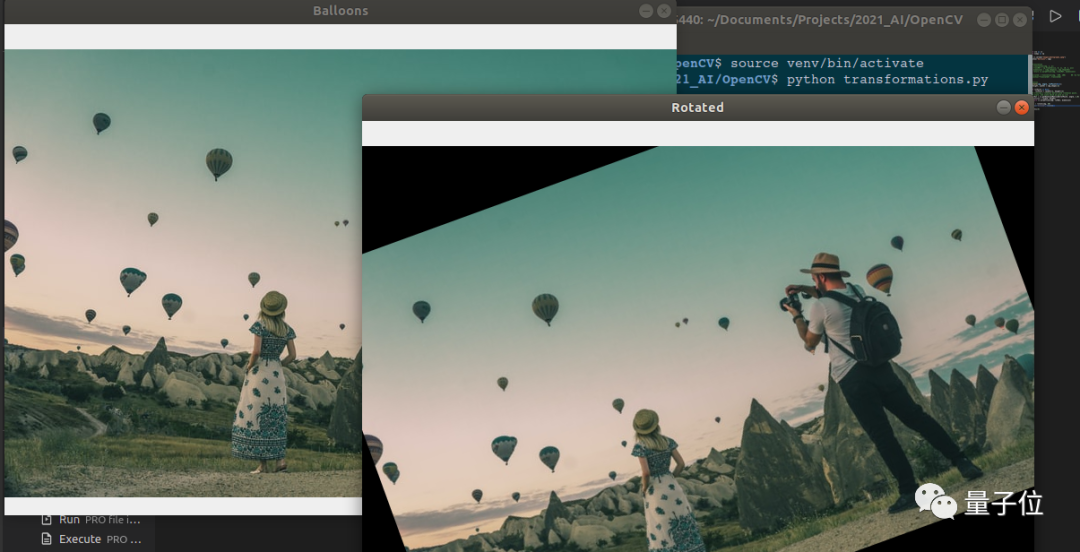
If you are interested in getting the job done simply and fast, read on. With the exception of cv2.INTER_LANCZOS4 - all methods have comparable running time.īut cv2.INTER_CUBIC And cv2.INTER_LINEAR slower than cv2.INTER_AREA And cv2.INTER_NEARESTbut cv2.INTER_LANCZOS4 is the slowest of all algorithms.If you are absolutely intent on doing this in Python, then please just disregard my answer. To zoom in, the best methods are (in order of decreasing speed): cv2.INTER_LINEAR, cv2.INTER_CUBIC, cv2.INTER_LANCZOS4. To zoom out, the best method is cv2.INTER_AREA. The resulting graphical representation of the speed of the algorithms: Let’s run the rescaling procedure 50 times with different scaling factors and different interpolation algorithms: n = 50įactors = Ĭv2.resize(img, None, fx=factor, fy=factor, interpolation=alg)ĭata.append(dict(time=mtime, algorithm=alg, error=err, scale=factor))Īx = df.set_index('scale').groupby("algorithm").plot(legend=True, figsize=(10, 8), grid=True, title="Algorithm time compare") In addition, we will consider a comparison of the speed of operation of various interpolation algorithms. Resize size: 384 448 Speed comparison of various interpolation methods when scaling images in OpenCV Img_up2 = resize_test(img_down2, 8, file_name="opencv_resize_up3.png") Img_up1 = resize_test(img_down1, 8, file_name="opencv_resize_up2.png") Img_up0 = resize_test(img_down0, 4, file_name="opencv_resize_up1.png") Img_down2 = resize_test(img3, 0.125, file_name="opencv_resize_down3.png") Img_down1 = resize_test(img2, 0.125, file_name="opencv_resize_down2.png") Img_down0 = resize_test(img, 0.25, file_name="opencv_resize_down1.png") Img_r = cv2.resize(img, (width2, height2), interpolation = alg) Height2, width2 = int(height*factor), int(width*factor) Testing: def resize_test(img, factor, is_plot=True, file_name=None): Set the types of interpolation: interpolation_algorithm = [ Img3 = cv2.cvtColor(imgorig3, cv2.COLOR_BGR2RGB) Img2 = cv2.cvtColor(imgorig2, cv2.COLOR_BGR2RGB) Img = cv2.cvtColor(imgorig, cv2.COLOR_BGR2RGB) Loading test images: imgorig = cv2.imread('cat4.jpg') Sns.set_context('notebook', rc=, font_scale=1.5) We connect the necessary libraries: import numpy as np Examples are shown for both upscaling and downsizing the image. Comparison of how various interpolation methods work when scaling images in OpenCVīelow are the results of the interpolation algorithms available in OpenCV when scaling images. It remains to compare the work of various interpolation methods in order to check in practice which one is better suited for reducing or enlarging images. * to enlarge the image – the most preferred interpolation methods: cv.INTER_CUBIC (slow) and cv.INTER_LINEAR. * for image compression - the preferred interpolation method is cv.INTER_AREA, In the official OpenCV tutorial ( Geometric Transformations of Images) states that: To choose a method – you need to understand – which of these methods is better?īy default, interpolation is performed by the method cv.INTER_LINEAR. The interpolation parameter sets one of several possible interpolation methods:Ĭv.INTER_NEAREST – interpolation by the nearest neighbor method (nearest-neighbor interpolation),Ĭv.INTER_LINEAR – bilinear interpolation ( used by default),Ĭv.INTER_CUBIC – bicubic interpolation (bicubic interpolation) in the vicinity of 4 × 4 pixels,Ĭv.INTER_AREA – resampling using the pixel area ratio,Ĭv.INTER_LANCZOS4 – Lanczos interpolation in the vicinity of 8 × 8 pixels.
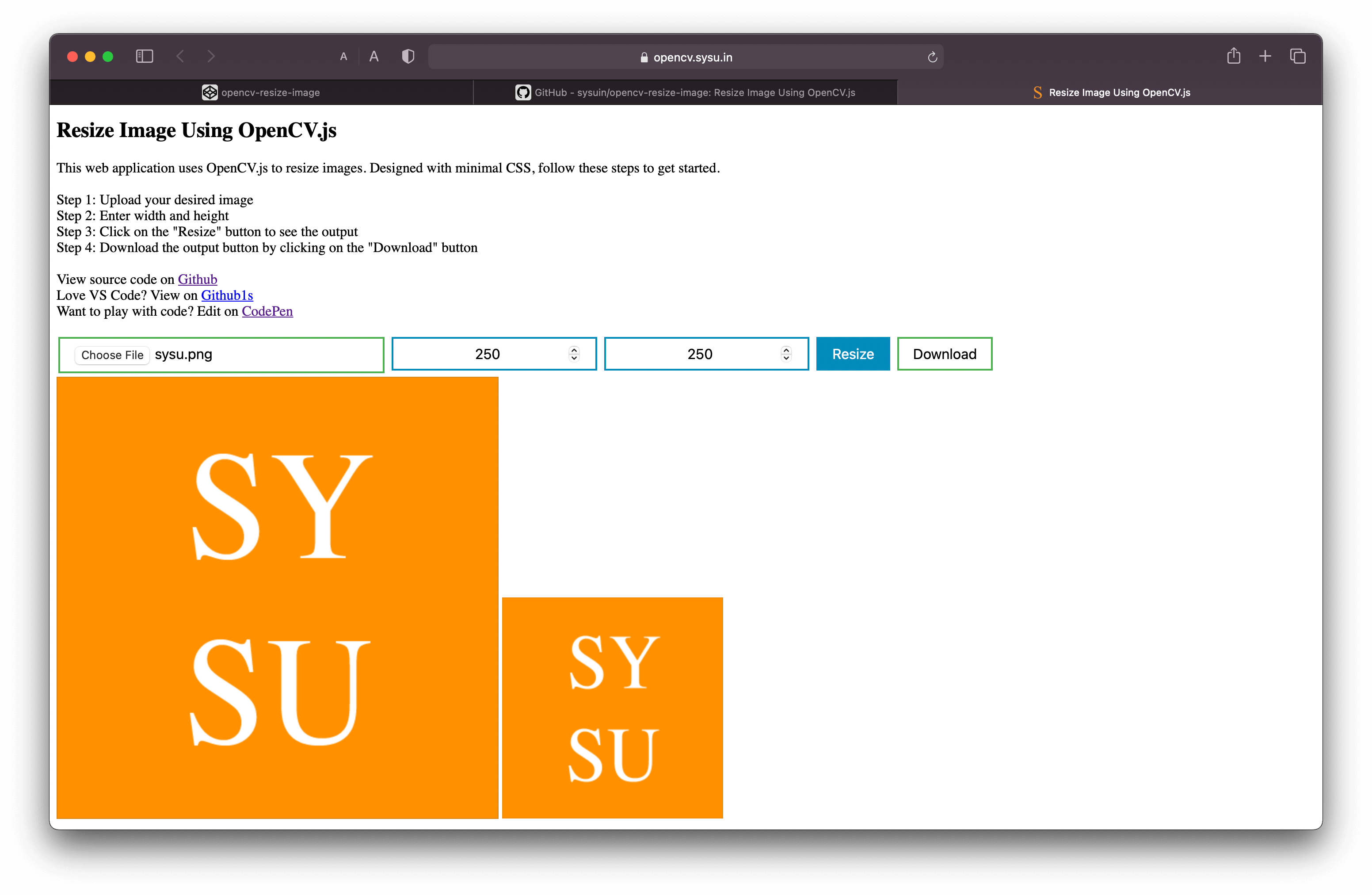
Res = cv.resize(img, None, fx=2, fy=2, interpolation = cv.INTER_CUBIC)
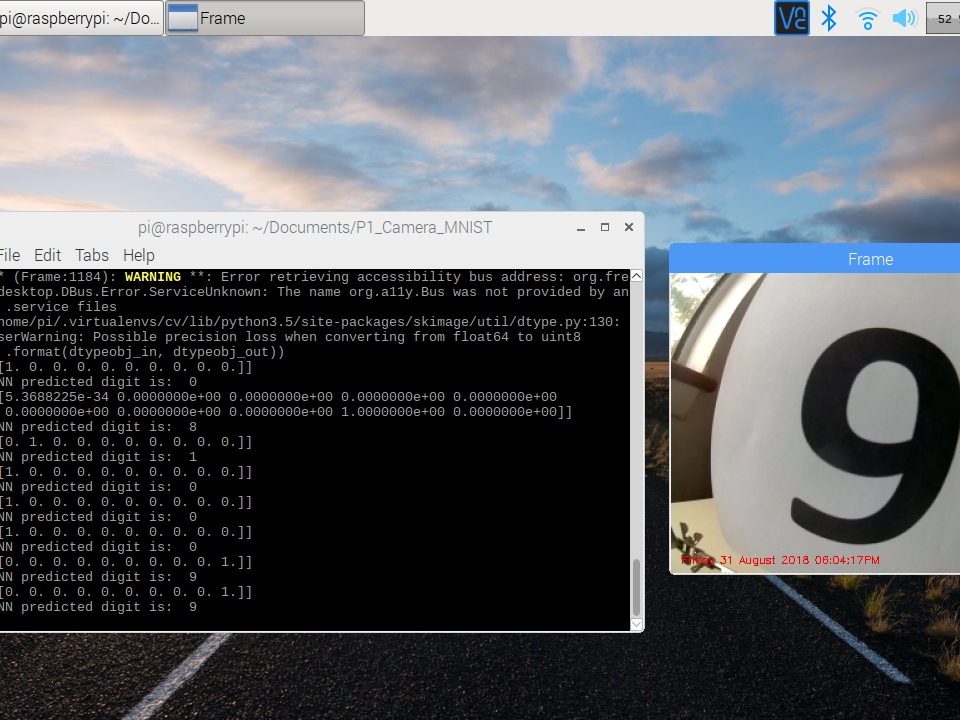
Res = cv.resize(img, (2*width, 2*height), interpolation = cv.INTER_CUBIC) The image size can either be specified manually (dsize), or scaling factors can be passed along the corresponding image axes: fx, fy. In Python: dst = cv2.resize( src, dsize]]] ) In C++: void resize(InputArray src, OutputArray dst, Size dsize, double fx=0, double fy=0, int interpolation=INTER_LINEAR ) OpenCV uses the function to accomplish this task resize(). Resizing (scaling / scaling) is a very commonly used method when working with images.
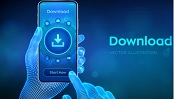